
Walk into a lower-middle-class suburb in New Delhi. Enter a chemist’s shop. Invariably, the chemist will not be a qualified pharmacist. Within minutes, you shall observe a poor or lower-middle-class patient walk in. He shall describe his symptoms. The chemist shall dispense what could be a lifesaver or a volatile cocktail of medicines comprising, in all probabilities a mix of, antibiotics, steroids, and painkillers. With experience, the chemist’s brain has evolved some kind of Expert System. Dispensing drugs based on thousands of prescriptions over the years, he has developed a small Neural Network Learning model based on which he considers himself qualified to treat patients. This article is about the developing world, however I shall use some data from India as an example.
In a recent study, “Two Indias: The Structure of Primary Health Care Markets in Rural Indian Villages with Implications for Policy,” by Jishnu Das and others published by Elsevier in 2020, as well as scores of other reports, highlight the following :
About 75% of villages have at least one health provider, 86% are in the private sector within the private sector, and the majority are ‘informal providers without any formal medical training. Such informal providers account for 68% of the private sector.
The informal providers use a combination of allopathic, ayurvedic, local medicine, and homeopathy. The quality of essential knowledge and upgradation of skills is highly suspect.
Primary healthcare centers are woefully short of qualified medical professionals and staff. There are about 5-6 healthcare workers per 10,000 in India compared to 44.5, as suggested by WHO. Most healthcare workers prefer to work in towns and cities.
Healthcare worker salaries/ stipends in Govt Primary Health Care centers are very low, therefore the number of pending vacancies is high.
The Medical Council of India regulates education in the Medical Sector. We produce about fifty thousand doctors per annum and inadequate healthcare workers. As per Niti Ayog, India has 11.5 Healthcare workers per 10,000 population vis a vis a requirement of 44.5 Healthcare workers needed in 2030 to meet Sustainable Development Goals suggested by WHO. With the present Medical College infrastructure these targets are impossible to meet.
Various suggestions to introduce education and certification for intermediate healthcare workers have been shot down over the decades. Millions shall suffer and die in the meantime.
Each developing/underdeveloped country has its dynamics; however, there must be many similarities between India and other developing nations.
AI-based Primary Healthcare is manna from heaven for such situations. Let us investigate. As per the authoritative “State of AI Report,” in 2022-23, the maximum investment in AI amounting to US$ 112 Billion was in the health sector, followed by Fintech and Enterprise!
It’s not cause for surprise. In the Western world, the USA alone spends US$ 3.5 Trillion ( equal to India’s present GDP) on Healthcare for a population of 334 million. The investment priorities of the West are, however, different. As per a report submitted in 2023 jointly by Science.org ( AAAS) and Icahn School of Medicine, Mount Sinai, USA, titled “Frontiers of Medical Research: Artificial Intelligence,” research and commercialization of AI shall happen in:
- Omics + AI in basic biomedical research
The use of ML/DL ( Machine Learning and Deep Learning)
Recent breakthroughs in the field of artificial intelligence (AI) are for
example, generative adversarial networks (GANs) or large language models (LLMs) and their application to advance basic biomedical research. Transformative advances are happening across all essential biomedical research subfields, including structural biology, genomics, systems biology, pharmacology, immunology, neuroscience, and developmental biology. Generative models can automatically design novel molecular entities, such as small molecules or proteins. Another area is the stratification and classification of patients based on clinical omics profiling. These methods can discover personalized disease biomarkers and novel targets faster and more effectively than genomics profiling alone.
2.0 Drug discovery. This is a capital-intensive and risky endeavor sucking up billions of US$ and years in research and approvals. Pharma companies generally spend an average of 20% of their revenues on R&D with foggy visibility on success, which is why the pharma industry is embracing AI. Recent deals by Astra Zenca, Merck, Sanofi, and BioNTech indicate that deal sizes are in the high hundred US$ Million range.
3.0 Pathology diagnostics and analysis.
While sophisticated equipment is used, much human intervention and judgment are involved, leading to errors. AI-based tools shall reduce such mistakes, providing better diagnosis and health care.
4.0 Segmentation of medical images.
Medical image segmentation is pivotal in modern Healthcare, enabling accurate and efficient diagnosis, treatment planning, and disease monitoring. Deep learning–based approaches have emerged as state-of-the-art due to their ability to learn complex features and spatial relationships from large datasets.
- Foundation visual models for radiology
Foundation models hold immense potential for revolutionizing the field of
radiology and Healthcare at large. The need for more open-source and large-scale datasets has been a limiting factor in medical imaging and initiatives. Furthermore, integrating multimodal data sources—medical imaging, electronic health records, and clinical reports—can significantly enhance the functionality and impact of these foundation models. Not only could they improve diagnostic accuracy, but they also have the potential to streamline clinical workflows, reduce patient burdens, and enable the delivery of precision medicine. - Weakly supervised learning for cancer detection in pathology images
Pathology is the most data-intensive modality in Medicine. Each image
displays tissue at the microscopic level with sub-cellular resolution, resulting in hundreds of thousands of pixels in each dimension. Using AI for pixel-level annotations is unnecessary; slide-level information can be used instead.
6. Digital health technologies, like wearables and integration with AI
Remote patient monitoring (RPM) leverages wearable devices to monitor
health and disease. It tracks blood glucose, blood pressure, cardiac rhythms, and mobility after surgery. It extends the ability of medical professionals to monitor individuals remotely. Monitoring often relies on connected devices tailored to a disease. RPM has improved health outcomes. For example, continuous glucose monitoring in type 1 diabetes mellitus results in improved glycemic control.

CB Insights, a data provider, estimates that investments in digital-health startups has nearly doubled in 2021 to $57bn. Unlisted healthcare startups valued at $1bn or more now number 90, four times the figure five years ago. Such “unicorns” are competing with incumbent healthcare companies and technology giants to make people better and prevent them from getting ill in the first place.

General Information- How does AI work?
Artificial intelligence (AI) is transforming the world. AI is also not the fad of the day but a result of the digitization of our civilization and a function of the resulting explosion of many different forms of data. The only way to handle this wealth of information is large-scale machine learning. In no other area is this more pertinent than in Healthcare, which produces more data than finance and entertainment combined.
Machine Learning (ML) is the discipline-focused on developing algorithms and systems that can learn from data. One subfield of machine learning is deep learning, which focuses on deep artificial neural networks with many layers, resulting in millions or billions of parameters, usually trained using backpropagation. Common deep learning architectures are Convolutional Neural Networks (CNNs), used mainly in computer vision, and attention-based Transformer models initially designed for sequential data, like language. Today, 99% of all AI is based on deep learning.
Recent advancements in generating coherent text and realistic images have led to the term Generative AI, which encompasses most ML models capable of generating samples from their underlying distributions.
Machine learning models can be trained using two approaches. The first approach is Supervised Learning, in which each sample is associated with a label. This label could be an image caption or an annotated CT scan. The second approach is Unsupervised Learning, which aims to find structure in the data without labels or annotation. This training technique is cost-effective as it does not rely on costly data annotation.
The first step in building machine learning models is to create Foundation Models. These models are then used in a second step to perform various tasks on smaller datasets that have already been labeled. Foundation Models can generate new samples and can also be used to convert input text or images into a low-dimensional vector space. Larger foundation models can accurately capture their underlying modality, meaning that no training or very few examples may be required for specific downstream tasks.
Much of the attention on early LLM ( Large Learning Models) applications in health care has been placed, for example, on their ability to pass the US medical licensing exam. Multimodal AI is a more profound analytic challenge. So far, there has been the integration of a few layers of data, such as electronic health records and genomics. Still, more is needed to approach the complexity, depth, and breadth of what can be relevant and analyzed.
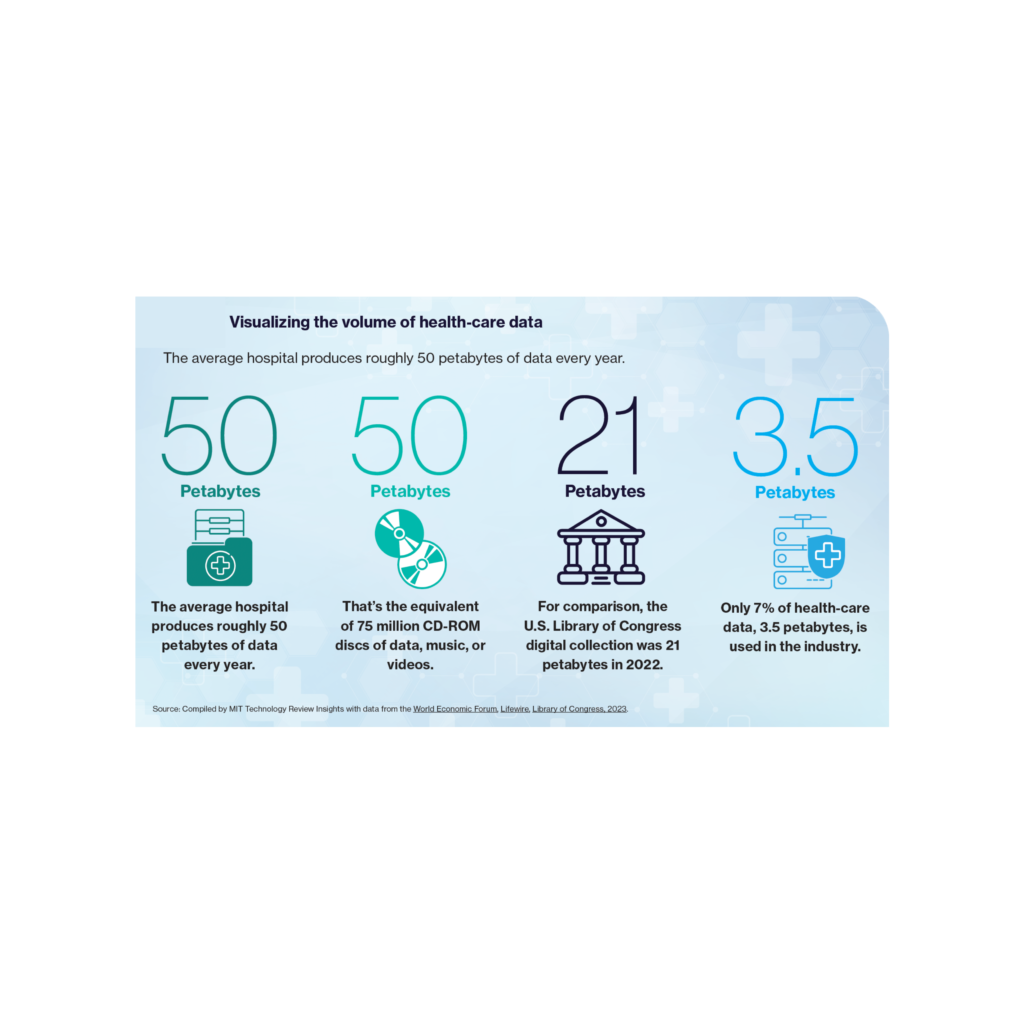
Large Learning Models are data-hungry. As per an estimate by MIT Technology Review Insights, in 2023, with data iku, a hospital in the USA can produce up to 20 petabytes of data- equivalent to 75 million CD-ROMs. The US Library of Congress’s digital collection in 2022 was just 22 petabytes. Presently, the industry is using only 3.5 petabytes of data. The National Health Service of the UK wanted to share their digital data for an LLM, to find with disappointment that most of it could not be used due to varying data fields and formats. For AI to succeed in Healthcare, standard data formats shall have to be agreed upon between various countries. Developing countries, unfortunately, have minuscule Electronic Data Records for Healthcare, and that is a significant constraint for creating local LLMs. Disease, after all, has geographical, spatial, and temporal relationships.
What are the latest developments in AI-based healthcare diagnostic applications? How can developing nations benefit from these technologies?
A number of companies are working on Mobile Applications for Disease Diagnostics. Healthcare–related AI interventions can be broadly divided into three application areas. The first includes AI-powered low-cost tools running on smartphones or portable instruments. These mainly address common diseases and are operated by nonspecialist informal health workers. Informal Healthworkers may use AI recommendations to triage patients and identify those requiring close follow-up. Applications include diagnosing skin cancer from photographic images and analyzing peripheral blood samples to diagnose malaria; more are expected given the emergence of pocket diagnostic hardware, including ultrasound probes and microscopes. With increasing smartphone penetration, patient-facing AI applications may guide lifestyle and nutrition, allow symptom self-assessment, and provide advice during pregnancy or recovery periods—ultimately allowing patients to take control of their health and reducing the burden on limited health systems.
The second application area focuses on more specialized medical needs, with the goal of supporting clinical decision-making. AI may allow nonspecialized primary care physicians to perform technical tasks, including reading diagnostic radiology and pathology images and only referring to specialists if necessary. AI tools may also help provide specialists with expert knowledge across multiple sub-specialties. This is particularly important in oncology, where a lack of subspecialists may force an oncologist to manage tumors across various anatomical sites, thus delivering care of inferior quality owing to the constantly varying scope of services.
The third application area relates to population health and allows public agencies to realize cause-and-effect relationships, appropriately allocate the often limited resources and ultimately mitigate the progression of epidemics. Improving data collection is central to these applications. Other applications include identifying hotspots for potential disease outbreaks in unmapped rural areas by utilizing AI-powered analysis of aerial photography and weather patterns.
AI applications in health care are far from perfect. Most are at the proof-of-concept stage and require further demonstration of utility through clinical validation in prospective trials. Data used to train AI models are skewed toward certain diseases, demographics, and geographies. With varying degrees of statistical data analysis and quality control, errors and systematic biases are introduced into models, limiting their generalizability, especially when deployed in different contexts. From a regulatory perspective, medical malpractice and liabilities in health-related algorithmic decision-making are yet to be formulated. Nearly all AI tools in health care are single-task applications, and so they are incapable of entirely substituting for health professionals.
Some applications of AI in Healthcare are:
IBM Watson Health, Google DeepMind Health, Zebra Medical Vision, Butterfly Network, Aidoc, Tempus, PathAI, Gauss Surgical, OWKIN and Prognos. However, these are rather specialized for certain functions except for Prognos.
Two AI Primary Care Healthcare Applications Suitable for the Developing World, including India! Challenges? Yes, but they can be overcome!
Ada a Germany based startup announced its push into the AI chatbot space quite some time back, and has consistently been funded in the last three years.
“There are different types of diagnostic tools out there,” says David Wong, a lecturer in health informatics at the Leeds Institute of Health Informatics. Wong, along with colleague Hamish Fraser, helped test the most popular artificial intelligence-powered symptom checker apps: Ada, Your.MD, and Babylon.
“Ada was by far the best,” says Wong. “There were issues with both of the others. It was surprising to be able to find things wrong in a few minutes, from a non-clinical perspective.”
Ada was also selected as the winner of WIRED’s test. It asks about the most important symptoms and provides the best diagnoses. The questions are clear, and it translates free text into sensible suggestions for the user to choose.
“From the beginning, we started building something that has a deep medical knowledge that covers rare conditions as well as common straightforward conditions,” says Ada founder Dr. Claire Novorol. She explains that Ada started out as a platform service for doctors and was then altered to focus on the bits patients could understand. “We’re now a platform connecting patients and doctors. In the UK, at the end of the assessment, you can share your assessment with a doctor, who is assisted by Ada’s doctor-facing technology on their side of the platform.”
Ada is open to country-specific localization and is free to use at this moment.
Your.MD
Wong says it wasn’t possible to get You.MD to diagnose shingles. “It did ask about whether I had previously had chickenpox and diagnosed related meningitis,” he says; it did provide the correct advice to see a GP. It correctly got to asthma and alcohol-related liver disease but failed with the UTIs, suggesting other sexually transmitted conditions instead” says Wong.
Significant developments are in the pipeline. Med-PaLM, developed by DeepMind, the AI firm owned by Alphabet, is another Large Language Model. Its 540bn parameters have been trained on data sets spanning professional medical exams, medical research, and consumer healthcare queries. Google’s Med-PaLM 2 language model is the first model to exceed a “passing” score on the US Medical Licensing. Examination (USMLE). In a pairwise ranking study on 1,066 medical questions, Med-PaLM 2 answers were preferred over physician answers by a panel of physicians across eight of nine axes in an evaluation framework.
Next Steps?
Developing countries have a major Primary Healthcare challenge. No amount of money can create needed Healthcare professionals by 2030. Governments of the day are quite helpless. Economic progress cannot be made with a sick population. Technology can be the enabler.
Cellular phone penetration in most developing countries is on the rise; smartphones are available in the remotest corners while 5G technology is being rolled out. AI – Healthcare Applications, in their present form can be used.
Informal Healthcare workers need to be recognized as an important part of Healthcare delivery. Extension work to help them upgrade skills and provide science based cure should be a priority. This is a win win for the patient and Informal Health Care provider.
None of the AI Healthcare tools however provide a Medicine Prescription with prices. AI Healthcare applications should be able to generate a Medicine Prescription which can be shared with the patient and chemist through a mobile phone.
As per law, only a certified pharmacist can dispense Medicine. However, this law is loosely interpreted and not applied; hence, getting Medicine from a local chemist should be possible.
AI applications should have a bare-bone Electronic Health Record system accessible to the patient and the health system.
Most chemists use low-cost IT systems for inventory management and generate bills with patient data and Medicines dispensed. The Government can make it mandatory for this data to be uploaded daily in a standard format and anonymize patients’ names and addresses.
This database in 2-3 years, could be the source to generate a Large Learning Model that is local and relevant.
The database should be available to any AI Healthcare technology provider free of charge to develop generic Monolithic or specific LLM’s.
The Government should introduce legislation for mandatory maintenance of health records in a unified format by all stakeholders in the health sector, to be uploaded on Govt managed servers.
Thank full acknowledgment to Science.org ( AAAS) and Icahn School of Medicine, Mount Sinai, USA for use of illustrations.
Leave a Reply